The High Tech Law Institute at Santa Clara Law, a co-sponsor of the AI for Social Impact Speaker Series, reprints this article with permission of the author, Steven Adler, whose original post can be found at Medium.
This week I had the great pleasure of meeting DJ Patil, Chief Data Scientist of the Obama Administration and former Chief Scientist at LinkedIn. Below is my summary and light analysis of his talk, which covered data science broadly (though I found quite relatable to AI ethics).
For further detail, I highly recommend DJ and his co-authors’ (Hilary Mason and Mike Loukides) excellent and free e-book, “Ethics and Data Science”, available here. Many thanks to the Markkula Center for Applied Ethics at Santa Clara University and other hosts for helping to convene this discussion, as part of their AI for Social Impact Speaker Series.
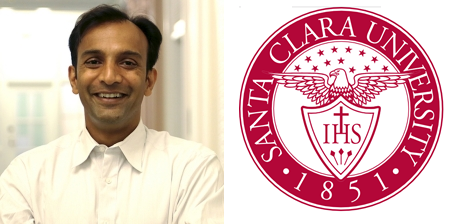
The speaker and hosts of the event, “ How to Do Good AI and Data Science (By Living Your Ethics)” with DJ Patil
Key questions for AI ethics/data ethics
- What standards and practices ought to be promulgated across AI and data science, as they grow in importance as fields?
- What mechanisms exist for promulgating these standards and practices, from the lens of a country/regulatory body, institution (such as a large for-profit or university), and individual?
- NB: I think the questions about governments and institutions are quite interesting, and deservedly getting much attention. Because the individual question is perhaps rarer and also more directly actionable, I add further color on it below.
Frameworks for understanding and managing AI/data science’s opportunities and challenges
DJ’s “5 Cs” — “a framework for implementing the golden rule of data” (treat others’ data as you’d like your own data to be treated; what dimensions do people care about when sharing their personal data?)
- Consent — Informed agreement on what data is to be collected and to what end; notions of consent and its limits can vary by geography (e.g., EU and GDPR).
- Clarity — Even when there appears to be consent, how confident are we that end-users understand the terms? This can be affected by technical complexity of usages and by legalese documents, such as form Terms and Conditions.
- Consistency (and trust) — Ensuring systems operate as expected and are reliable, so that users have accurate mental models of how their data will be used and the data-holder’s responsibilities.
- Control (and transparency) — Understanding what data is held, on what dimensions, and to what use, on an ongoing basis.
- Consequences — Anticipating how data might be misused or abused, either by malevolent actors or by well-intentioned actors with unforeseen circumstances.
DJ’s “data science project checklist” — what are the questions a data science team should ask before undertaking or launching a project, akin to other product review questions a team may undergo (e.g., will this new launch cannibalize our existing products)
- Have we listed how this technology can be attacked or abused?
- Have we tested our training data to ensure it is fair and representative?
- Have we studied and understood possible sources of bias in our data?
- Does our team reflect diversity of opinions, backgrounds, and kinds of thought?
- What kind of user consent do we need to collect to use the data?
- Do we have a mechanism for gathering consent from users?
- Have we explained clearly what users are consenting to?
- Do we have a mechanism for redress if people are harmed by the results?
- Can we shut down this software in production if it is behaving badly?
- Have we tested for fairness with respect to different user groups?
- Have we tested for disparate error rates among different user groups?
- Do we test and monitor for model drift to ensure our software remains fair over time?
- Do we have a plan to protect and secure user data?
I should note as well here that while cybersecurity is directly present in a few of the above questions (for instance, in questions 8, 9, and 13), this was also a central focus of DJ’s talk, e.g., in describing ‘red-team’ approaches to both infiltrating data systems and to how systems may be misused or abused.
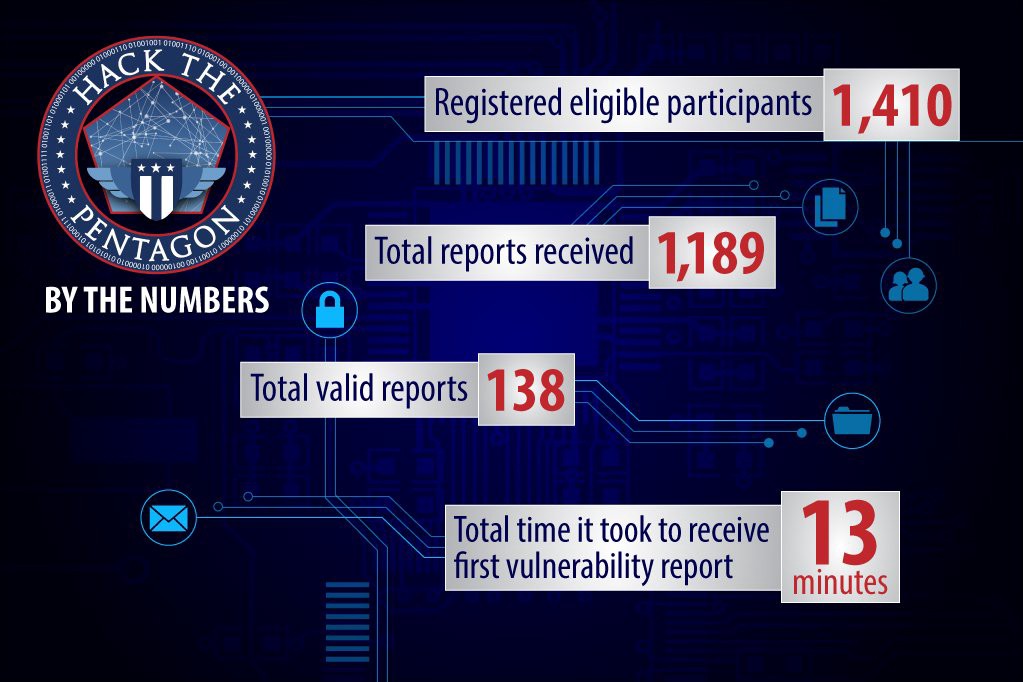
DJ highlighted the “Hack the Pentagon” program as a success of red-teaming and bringing cybersecurity into the realm of data science — even within a highly-confidential organization.
DJ’s suggestions for individuals
Given DJ’s perspective and his work across the data science ecosystem, what can one start doing today that might have an impact?
- If working on a data science project, take a look at the checklist outlined above. Could your team use this? If not, what questions need to be added or subtracted to make it more tenable?
- Ask yourself: If, in the course of a project, you were to run into a challenge with the 5 Cs or a question from the checklist, do you know the resolution mechanism? If not, can you speak with your manager or team about formalizing a process (including, perhaps, a neutral third-party mediator/ombudsperson)?
- When interviewing candidates to join your team, can you screen for amount of thought given to ethical tradeoffs, and ensure applicants are aligned with the vision you’d like your team to embody? (Where do AI and data ethics fit into your team’s notion of cultural fit?)
- When interviewing as a candidate at companies, can you ask them how they resolve data ethics challenges internally, and how they ensure their products are used to good ends? (DJ raised a good point here that if this question became common practice, you’d certainly see more activity around the field — this ties into the bottom-up, worker-demand side of the economic equation I allude to below.)
- When things go wrong (or narrowly avoid going wrong) in a project, does your team already have a post-mortem process for analysis? If not, can you suggest one oriented around the checklist above? (How can your team ensure appropriate fact-finding in the short-term and proper accountability in the long-term to help inform these practices?)
One additional framework for changing AI/data science ethics
An economic lens — though this framework was not called out directly, I view it as an essential theme in DJ’s talk and in my conversations with other participants — how to alter market dynamics (both supply- and demand-side) such that practicing strong data ethics becomes the norm.
Of course, this perspective is an oversimplification of the realities of institutional life and decision-making. At the same time, I think it is a useful oversimplication for considering how change may come, and where collective action may help.
As a starting point, I believe that nearly all AI scientists and adjacent stakeholders want very badly to do the ‘right’ thing — both deploying technologies toward great ends and avoiding misuses along the way.
Despite these inclinations, individuals within institutions can sometimes deviate from ideal behavior, based in part on lacking core tools to decisively answer the ethical questions, and in part from their own economic challenges and difficult incentive-structures.
To bring systemic change, therefore, we may find greater success when we couch practical solutions within people’s and institutions’ economic realities and ensure that ethics and market pressures function compatibly.
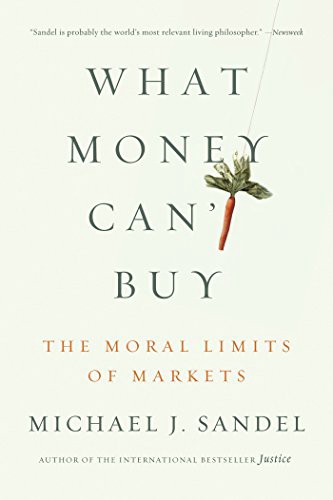
Market outcomes are not guaranteed to have been ethical — but aligning ethics with market incentives may make for an easier case for action.
Consider a hypothetical individual at a company, who is evaluated principally by the output of code or product features they ship, without an ethical evaluation factoring in; this individual will have good reasons to overlook gray areas that would delay their practices, particularly if flagging these issues may not even result in clear resolution.
“Pulling the production-line cord” and halting a project’s progress may not be valued by the company (and in turn by the individual) if these traits do not show up positively in one’s reviews. Individuals need to feel empowered and actively encouraged to take such actions — not just that these actions are permissible. (I felt that my former employer, McKinsey & Company, really excelled on this front, emphasizing the obligation to dissent at all levels and trying to embody non-hierarchical decision-making.)
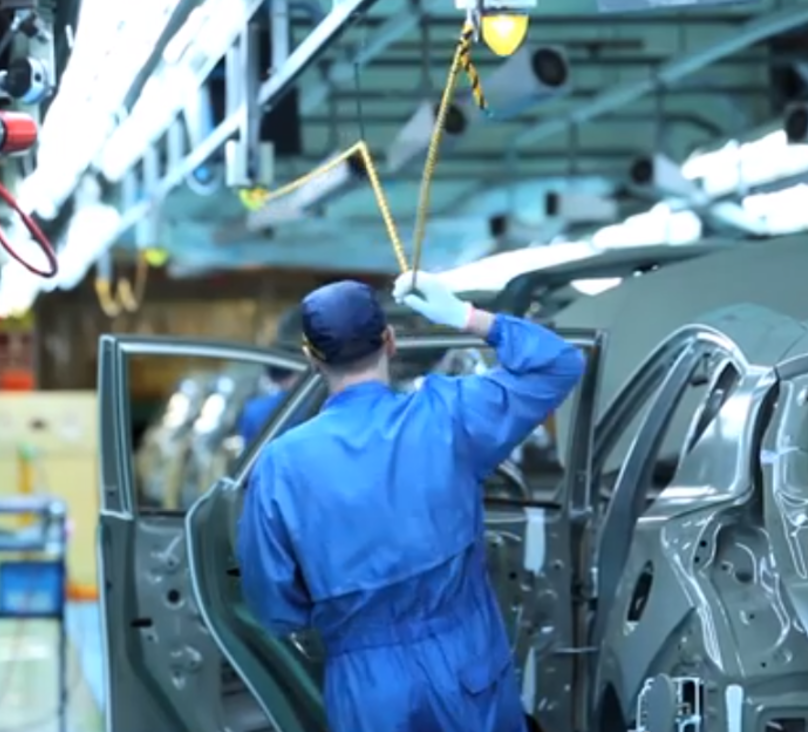
DJ highlighted Toyota’s use of “Andon Cords” in their manufacturing processes to empower any worker to stop production, should they see a sufficiently glaring issue. To encourage this conduct, companies must then reward such workers, rather than punish them as whistleblowers.
At an institutional level, I suspect that marketplace demands may encourage companies to continue launching AI/data science use cases, even if open questions remain about data usage, so long as the companies continue to be rewarded. (Fear of poor public perception, of course, is a powerful deterrent to hasty launches.)
Yet in practice, company policy is not as simple as deciding a priori what will maximize profits, and then magically willing it; there is an additional market dynamic at play. Within companies, well-positioned and ideologically-aligned individuals may be able to affect launch trajectory, particularly if they have unique skills or a lot of clout in the organization. These mechanisms of influence include by individually refusing to work on certain projects, or by helping to spark conversation against certain projects. In this sense, powerful workers with a strong sense for ethical guardrails can make a difference, at least within certain companies.
While individuals within companies can make attempts to steer the ship, and indeed often succeed, it remains a reality that many US companies do operate with a focus on shareholder value in many parts of their operations. A framework for AI/data science ethics, at least in the short-term, likely involves operating within that framework, or at least acknowledging its practicalities (say, couching objections in difficulties of recruitment, retention, or implementation feasibility should certain scientists not endorse a mission).
None of these solutions nor frameworks is a panacea; in some cases, they leave to-be-determined what specific ethics folks ought to embody (what is the vision your team is driving toward in its use of AI/data science, and from where does this hail), and in others they are smaller incremental change, rather than top-down directives that may eventually achieve more scale. (Though taken as a sum, many smaller-scale changes may amount to broader change, or may help to stoke larger-scale actors.)
Still, I found it both inspiring and empowering to leave DJ’s talk with a concrete set of actions one can take to “live their ethics” in AI and data science more generally. I am very grateful to have had this opportunity, and hope that my synthesis and thoughts are useful to others grappling with these questions.
Steven Adler is a former strategy consultant focused across AI, technology, and ethics.